Data Partitioning
Data partitioning, also known as sharding, is a technique used in distributed databases and systems to divide a large dataset into smaller, more manageable pieces, called partitions or shards. Each partition can be stored and processed independently across different nodes in a distributed system, improving scalability, performance, and manageability.
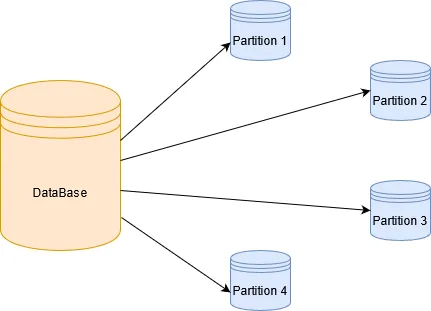
Why Data Partitioning?
- Scalability: As data volume grows, a single machine may not be able to store or process all the data efficiently. Partitioning allows the dataset to be distributed across multiple machines, enabling horizontal scaling.
- Performance: By distributing the workload across multiple machines, partitioning can reduce query response times and increase throughput.
- Manageability: Smaller datasets are easier to manage, back up, and restore compared to a single large dataset.
Types of Data Partitioning
- Horizontal Partitioning (Sharding): Divides the dataset into rows, distributing different rows across multiple partitions. Each partition contains a subset of the rows.
- Example: Splitting a customer database where customers with IDs 1-1000 are in partition A, and customers with IDs 1001-2000 are in partition B.
- Vertical Partitioning: Divides the dataset into columns, where each partition contains a subset of the columns.
- Example: Storing customer contact information (name, phone, email) in partition A, and customer account information (account number, balance) in partition B.
- Range-Based Partitioning: Divides data based on a continuous range of values.
- Example: Orders placed in January go to partition A, orders placed in February go to partition B.
- Hash-Based Partitioning: Uses a hash function to determine the partition for each piece of data.
- Example: Applying a hash function to customer IDs to evenly distribute customers across partitions.
- List-Based Partitioning: Divides data based on a predefined list of values.
- Example: Customers from the USA go to partition A, customers from Canada go to partition B.
- Composite Partitioning: Combines multiple partitioning strategies to create a more complex partitioning scheme.
- Example: First partitioning orders by range (month) and then by hash within each month.
Partitioning Strategy Considerations
Choosing the right partitioning strategy depends on several factors:
- Data Distribution: Ensure an even distribution of data to avoid hot spots where some partitions receive significantly more data and traffic than others.
- Query Patterns: Understand common query patterns to minimize cross-partition queries, which can be costly and reduce performance.
- Scalability Needs: Consider how the partitioning strategy will support future growth in data volume and query load.
- Maintenance: Assess the complexity of maintaining the partitioning scheme, including tasks like rebalancing data across partitions.
Challenges of Data Partitioning
- Data Skew: Uneven distribution of data across partitions can lead to performance bottlenecks.
- Cross-Partition Queries: Queries that span multiple partitions can be slow and complex to execute.
- Rebalancing: As data grows or query patterns change, rebalancing partitions may be necessary, which can be complex and resource-intensive.
- Consistency: Ensuring data consistency across partitions can be challenging, especially in distributed systems.